Publicación: Modelado de Propiedades Críticas de Sustancias mediante Redes Neuronales y Estructura Molecular
dc.contributor.advisor | López Santamaría, Camilo Andrés | |
dc.contributor.author | Mayorga Chaves, Eliana Marcela | |
dc.date.accessioned | 2024-07-11T20:40:33Z | |
dc.date.available | 2024-07-11T20:40:33Z | |
dc.date.issued | 2024 | |
dc.description.abstract | Este estudio se centra en la aplicación de redes neuronales en ingeniería química para la estimación precisa de propiedades críticas, como la presión y la temperatura crítica, fundamentales en el diseño de plantas químicas. En Colombia, la industria química enfrenta un desafío importante debido a la falta de avances tecnológicos en el diseño de equipos para sectores clave como agricultura, alimentos, farmacéutica y petroquímica. Actualmente, el diseño de equipos se basa en modelos empíricos o determinísticos que, carecen de la información necesaria para realizar estimaciones precisas de las variables pertinentes. Ante esta problemática, se propone el desarrollo de una red neuronal que se enfoca en la temperatura crítica como parámetro principal. Esta red utiliza matrices de distancia y conectividad para representar la información de la estructura molecular de las sustancias. Adicionalmente, usa descriptores como peso molecular y electronegatividad. Los resultados conllevan a estimaciones de propiedades críticas, especialmente para sustancias orgánicas, partiendo de matrices de conectividad. Este enfoque promete contribuir al avance tecnológico en la industria química colombiana al proporcionar herramientas más precisas y eficientes para el diseño de procesos y equipos. Además, abre nuevas oportunidades para investigaciones futuras en el campo de la química computacional y la inteligencia artificial aplicada a la ingeniería química con el fin de satisfacer las necesidades industriales y sociales. | spa |
dc.description.abstract | This study focuses on the application of neural networks in chemical engineering for the accurate estimation of critical properties, such as pressure and critical temperature, fundamental in the design of chemical plants. In Colombia, the chemical industry faces a major challenge due to the lack of technological advances in the design of equipment for key sectors such as agriculture, food, pharmaceuticals and petrochemicals. Currently, equipment design is based on empirical or deterministic models that lack the necessary information to make accurate estimates of the relevant variables. In view of this problem, we propose the development of a neural network that focuses on the critical temperature as the main parameter. This network uses distance and connectivity matrices to represent the molecular structure information of the substances. Additionally, it uses descriptors such as molecular weight and electronegativity. The results lead to estimates of critical properties, especially for organic substances, based on connectivity matrices. This approach promises to contribute to technological progress in the Colombian chemical industry by providing more accurate and efficient tools for process and equipment design. It also opens new opportunities for future research in the field of computational chemistry and artificial intelligence applied to chemical engineering to meet industrial and societal needs. | eng |
dc.description.degreelevel | Pregrado | spa |
dc.description.degreename | Tecnólogo en Procesos Químicos Industriales | spa |
dc.description.program | Tecnología en Procesos Químicos Industriales | spa |
dc.format.extent | 66 p. | spa |
dc.format.mimetype | application/pdf | spa |
dc.identifier.uri | https://repositorio.ecci.edu.co/handle/001/4080 | |
dc.language.iso | spa | spa |
dc.publisher | Universidad ECCI | spa |
dc.publisher.faculty | Facultad de Ingenierías | spa |
dc.publisher.place | Colombia | spa |
dc.relation.references | Ahirwar, H., Kurmi, G., Khan, R., Khare, B., Jain, A., Jain, P. K., & Thakur, B. S. (2022). Review on QSAR using Anticancer Drug. Asian Journal of Dental and Health Sciences, 2(4), 5963. https://doi.org/10.22270/ajdhs.v2i4.27 | spa |
dc.relation.references | Alander, J. T. (n.d.). On optimal population size of genetic algorithms. CompEuro 1992 Proceedings Computer Systems and Software Engineering, 65–70. https://doi.org/10.1109/CMPEUR.1992.218485 | spa |
dc.relation.references | Basheer, I. A., & Hajmeer, M. (2000). Artificial neural networks: Fundamentals, computing, design, and application. Journal of Microbiological Methods, 43(1), 3–31. https://doi.org/10.1016/S0167-7012(00)00201-3 | spa |
dc.relation.references | Bettina, M. (2011). ESTRATEGIA DE PARAMETRIZACIÓN DEL MODELO A-UNIFAC. https://repositoriodigital.uns.edu.ar/bitstream/handle/123456789/2295/GARRIGATesis.pdf?sequence=1&isAllowed=y | spa |
dc.relation.references | Bonilla, B., & Herrera, J. N. (2006). Revisando la ecuación de van der Waals. | spa |
dc.relation.references | Forero-Corba, W., & Bennasar, F. N. (2024). Techniques and applications of Machine Learning and Artificial Intelligence in education: a systematic review. RIED-Revista Iberoamericana de Educacion a Distancia, 27(1), 209–253. https://doi.org/10.5944/ried.27.1.37491 | spa |
dc.relation.references | García Díaz, E. E., & Lozano Martínez, F. (2006). Boosting Support Vector Machines. Revista de Ingeniería, 24, 62–70. https://doi.org/10.16924/revinge.24.8 | spa |
dc.relation.references | Jumper, J., Evans, R., Pritzel, A., Green, T., Figurnov, M., Ronneberger, O., Tunyasuvunakool, K., Bates, R., Žídek, A., Potapenko, A., Bridgland, A., Meyer, C., Kohl, S. A. A., Ballard, A. J., Cowie, A., Romera-Paredes, B., Nikolov, S., Jain, R., Adler, J., … Hassabis, D. (2021). Highly accurate protein structure prediction with AlphaFold. Nature, 596(7873), 583–589. https://doi.org/10.1038/s41586-021-03819-2 | spa |
dc.relation.references | Li, S., Dan, Y., Li, X., Hu, T., Dong, R., Cao, Z., & Hu, J. (2020). Critical temperature prediction of superconductors based on atomic vectors and deep learning. Symmetry, 12(2). https://doi.org/10.3390/sym12020262 | spa |
dc.relation.references | López de Castilla Vásquez, C. (2019). Redes bayesianas con algoritmos basados en restricciones, scores e híbridos aplicados al problema de clasificación. Anales Científicos, 80(1), 15. https://doi.org/10.21704/ac.v80i1.1370 | spa |
dc.relation.references | McMurry, J. (2016). Organic Chemistry. Cengage Learning . https://dl.iranchembook.ir/ebook/organic-chemistry-419.pdf | spa |
dc.relation.references | Ottow, E. (Eckhard), & Weinmann, Hilmar. (2008). Molecular Descriptors for Chemoinformatics. Wiley-VCH. | spa |
dc.relation.references | Prausnitz, J., Lichtenthaler, R., & Gomes de Azevedo, E. (2000). Termodinámica Molecular en Equilibrio de Fases. Isabel Capella. | spa |
dc.relation.references | Rayner Canham, G. (2000). Quimica Inorganica Descriptiva, 2da Edición. www.FreeLibros.me | spa |
dc.relation.references | Scalia, G., Grambow, C. A., Pernici, B., Li, Y. P., & Green, W. H. (2020). Evaluating Scalable Uncertainty Estimation Methods for Deep Learning-Based Molecular Property Prediction. Journal of Chemical Information and Modeling, 60(6), 2697–2717. https://doi.org/10.1021/acs.jcim.9b0097 | spa |
dc.relation.references | Stack, P. (2021). Métodos de Aprendizaje Supervisado y no Supervisado para la Estimación de Microestructura Cerebral en Datos de DWMR. Centro de Investigación En Matemáticas, A.C. https://cimat.repositorioinstitucional.mx/jspui/bitstream/1008/1129/1/TE%20835.pdf | spa |
dc.relation.references | Venkatasubramanian, V. (2019). The promise of artificial intelligence in chemical engineering: Is it here, finally? AIChE Journal, 65(2), 466–478. https://doi.org/10.1002/aic.16489 | spa |
dc.relation.references | Zavala, V. M. (2023). Outlook: How i Learned to Love Machine Learning (A Personal Perspective on Machine Learning in Process Systems Engineering). In Industrial and Engineering Chemistry Research (Vol. 62, Issue 23, pp. 8995–9005). American Chemical Society. https://doi.org/10.1021/acs.iecr.3c01565 | spa |
dc.relation.references | Zhang, L., Han, J., Wang, H., Car, R., & Weinan, E. (2018). Deep Potential Molecular Dynamics: A Scalable Model with the Accuracy of Quantum Mechanics. Physical Review Letters, 120(14). https://doi.org/10.1103/PhysRevLett.120.143001 | spa |
dc.rights | Derechos Reservados - Universidad ECCI, 2024 | spa |
dc.rights.accessrights | info:eu-repo/semantics/openAccess | spa |
dc.rights.coar | http://purl.org/coar/access_right/c_abf2 | spa |
dc.rights.license | Attribution-NonCommercial-NoDerivatives 4.0 Internacional | * |
dc.rights.uri | http://creativecommons.org/licenses/by-nc-nd/4.0/ | * |
dc.subject.proposal | Temperatura Crítica | spa |
dc.subject.proposal | Critical Temperature | eng |
dc.subject.proposal | Red Neuronal | spa |
dc.subject.proposal | Neural Network | eng |
dc.subject.proposal | Sustancias Orgánicas | spa |
dc.subject.proposal | Organic Substances | eng |
dc.subject.proposal | Sustancias Inorgánicas | spa |
dc.subject.proposal | Inorganic Substances | eng |
dc.title | Modelado de Propiedades Críticas de Sustancias mediante Redes Neuronales y Estructura Molecular | spa |
dc.title.translated | Modeling Critical Properties of Substances using Neural Networks and Molecular Structure | |
dc.type | Trabajo de grado - Pregrado | spa |
dc.type.coar | http://purl.org/coar/resource_type/c_46ec | spa |
dc.type.coarversion | http://purl.org/coar/version/c_970fb48d4fbd8a85 | spa |
dc.type.content | Text | spa |
dc.type.driver | info:eu-repo/semantics/other | spa |
dc.type.redcol | https://purl.org/redcol/resource_type/WP | spa |
dc.type.version | info:eu-repo/semantics/updatedVersion | spa |
dspace.entity.type | Publication |
Archivos
Bloque de licencias
1 - 1 de 1
Cargando...
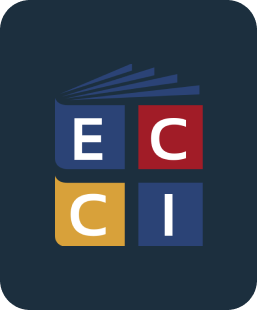
- Nombre:
- license.txt
- Tamaño:
- 14.45 KB
- Formato:
- Item-specific license agreed upon to submission
- Descripción: