Publicación: Creación de una herramienta de voz a texto utilizando un motor de software libre para facilitar la inclución digital en educacion telepresencial a la comunidad no oyente de la Universidad ECCI
dc.contributor.advisor | Sabogal Rueda, Alexander | |
dc.contributor.author | Duarte Cortes, Luis Alberto | |
dc.contributor.researchgroup | Sigestecci | spa |
dc.date.accessioned | 2023-08-15T14:45:22Z | |
dc.date.available | 2023-08-15T14:45:22Z | |
dc.date.issued | 2022 | |
dc.description.abstract | The purpose of this project is to develop a free software prototype tool for assisting hearing-impaired students that struggle with virtual academic environments. This comes from direct experience of the needs of this community using this platform during the Covid-19 restrictions where captions were unavailable during classes so hearing-impaired students required a full-time sign interpreter during classes to communicate accurately with the teacher. The main motivation for this development is to achieve easier adoption of new virtuality use cases, and solve the evident absence of this service since it is not provided by the university. This proposal is born from the Free Software Research hotbed from the ECCI University to dig deeply into voice-to-text technologies as a primary source to conduct a state-of-the-art analysis using different voice-to-text engines in search of the one most suitable for developing a functional prototype of a voice-to-text based web application that allows students and the university free access to a subtitle generation service that can be provided during conferences, speeches, and extracurricular activities. In this document, you will be able to visualize the different proposed phases: analysis, design, implementation, execution, and testing made to the web application in the last part of the document. The conclusions and contributions on further implementation will be reviewed, as well as recommendations for upgrading and scaling up this platform to be widely used by the community. | eng |
dc.description.abstract | En este documento se muestra el desarrollo de un prototipo de Software Libre para asistir a los estudiantes de la comunidad de discapacidad auditiva en ambientes virtuales, esto a partir de la observación directa de las necesidades especiales de esta población en estas plataformas durante las restricciones de COVID-19 donde los subtítulos de apoyo no estaban disponibles para ellos ya que dependen de un intérprete de lengua de señas para comunicarse adecuadamente con un docente. La motivación principal para desarrollarlo es lograr que los estudiantes sordos se adapten fácilmente a los nuevos usos de la virtualidad, y solucionar la evidente ausencia de este servicio por parte de la Universidad, Para esto se realizó desde el semillero de Software Libre de la Universidad ECCI una investigación a fondo sobre estas tecnologías de reconocimiento de voz como una investigación primaria a partir de la cual se elaboró un estado del arte analizando los diferentes motores de voz en busca del motor más adecuado para desarrollar un prototipo funcional de una herramienta de voz a texto basada en una aplicación web que le permita a los estudiantes y a la Universidad acceder a este servicio como apoyo visual de subtítulos en conferencias charlas y actividades académicas extracurriculares. En este documento se visualizan las diferentes fases planteadas: análisis, diseño, implementación, ejecución y pruebas realizadas a la aplicación web y en la parte final del documento, se evidencian las conclusiones y aportes sobre la investigación, así como recomendaciones para actualizar y escalar esta plataforma, para que sea ampliamente utilizada por la comunidad. | spa |
dc.description.degreelevel | Pregrado | spa |
dc.description.degreename | Ingeniero en Sistemas | spa |
dc.description.methods | Modelo Gavilán | spa |
dc.description.program | Ingeniería de Sistemas | spa |
dc.description.researcharea | Software Libre - Desarollo | spa |
dc.description.tableofcontents | 1. Título de la Investigación 15 2. Problema de la Investigación 16 2.1 Descripción del Problema 16 2.2 Formulación del Problema 20 3. Objetivos de la Investigación 21 3.1 Objetivo General 21 3.2 Objetivos Específicos 21 4. Justificación y Delimitaciones de la Investigación 22 4.1 Justificación 22 4.2 Delimitaciones 22 5. Marco de referencia 23 5.1 Marco Teórico 23 5.1.1 Reconocimiento de voz a texto. 23 5.1.2 Ambientes de ejecución 51 5.1.3 Infraestructura TI web 53 5.2 Marco Conceptual 60 5.2.1 Reconocimiento automático de voz 60 5.2.2 ASR 60 5.2.3 Plataforma 61 5.2.4 Web Socket 61 5.2.5 API 61 5.2.6 Bit-Rate 62 5.2.7 Características de voz 62 5.2.8 Características del lenguaje 64 Marco Legal 66 5.2.9 Leyes 66 5.2.10 Decretos 66 5.2.11 Circulares 67 5.2.12 Licencias de Software Libre 67 6. Ingeniería de Requerimientos 68 6.1 Acta Inicio del Proyecto 68 6.2 Fases de implementación 68 6.2.1 Metodología 68 6.3 Identificación de necesidades 73 6.4 Investigación Preliminar estado del arte 75 6.4.1 Modelo gavilán 75 6.4.2 Problema de investigación 78 6.4.3 Búsqueda y recolección de información 79 6.4.4 Análisis de la información obtenida 92 6.5 Selección del motor de Software 93 6.6 Especificación de requisitos de la infraestructura 96 6.6.1 Sistema Operativo 96 6.7 Especificación de requisitos del servicio 96 6.7.1 Servidores 96 6.7.2 Navegadores WEB 97 6.8 Diseño y elaboración de la propuesta 98 6.8.1 Diagramas y diseño 98 6.8.2 Infraestructura 105 6.8.3 Planimetría de Red 105 6.8.4 Descripción de servicio 108 7. Solución propuesta 109 7.1 Descripción de la propuesta 109 7.2 Desarrollo de la propuesta 110 7.3 Instalación de Software principal 110 7.4 Pruebas Realizadas a la propuesta 111 7.4.1 Entorno de pruebas locales 111 7.4.2 Entorno de despliegue 113 7.4.3 Pruebas 115 7.5 Análisis de Resultados Obtenidos 119 7.6 Acta Cierre del Proyecto 119 8. Recursos 120 8.1 Recursos Humanos 120 8.1.1 Líder de proyecto 120 8.1.2 Director de proyecto (Universidad ECCI) 120 8.1.3 Asesor de proyecto (Universidad ECCI) 120 8.2 Recursos Físicos 120 8.3 Recursos Tecnológicos 120 8.3.1 Servidor de pruebas 120 8.3.2 Servidor de despliegue 121 9. Cronograma de Actividades 121 10. Conclusiones 121 11. Bibliografía 123 | spa |
dc.description.technicalinfo | se incluye documentacion configuracion y ejecucion de la plataforma en el ANEXO 10 junto con la plataforma y el producto de desarollo en el ANEXO 11 | spa |
dc.format.extent | 137 p. | spa |
dc.format.mimetype | application/pdf | spa |
dc.identifier.uri | https://repositorio.ecci.edu.co/handle/001/3546 | |
dc.language.iso | spa | spa |
dc.publisher | Universidad ECCI | spa |
dc.publisher.faculty | Facultad de Ingenierías | spa |
dc.publisher.place | Colombia | spa |
dc.relation.references | Alpha Cephei. (15 de Marzo de 2022). Vosk. Obtenido de https://alphacephei.com/vosk/ | spa |
dc.relation.references | Amazon. (15 de Agosto de 2022). ASR AWS. Obtenido de Pricing: https://aws.amazon.com/transcribe/pricing/?nc=sn&loc=3 | spa |
dc.relation.references | appareo. (29 de abril de 2021). AVIATION SPEECH RECOGNITION SYSTEM. Obtenido de Aviation Speech Recognition System Using Artificial Intelligence: https://appareo.com/aviation/aviation-speech-recognition-system/ | spa |
dc.relation.references | Beazley, D. M. (2009). Python Essential Reference. Addison-Wesley Professional. | spa |
dc.relation.references | Bermuth, D. a. (2021). Scribosermo: Fast Speech-to-Text models for German and other Languages. arXiv preprint arXiv:2110.07982. | spa |
dc.relation.references | Bloch, J. (2018). A Brief, Opinionated History of the API. QCon (pág. 1). San Francisco: Enterprise Software Development Community. Obtenido de https://www.infoq.com/presentations/history-api/ | spa |
dc.relation.references | Bolaños Araya, C., Camacho Lozano, A., & Urrutia, X. d. (2017). USO DE LA ENTONACIÓN PARA IDENTIFICAR CUÁNDO USAR LA TILDE DIACRÍTICA EN EL RECONOCIMIENTO AUTOMÁTICO DEL HABLA. Káñina, 40(4), 13. Obtenido de https://doi.org/10.15517/rk.v40i4.30222 | spa |
dc.relation.references | Botti, V., & Serra, J. M. (2001). Aplicación de una red neuronal para la predicción de la reacción catalítica isomerización del n-Octano. Valencia: Universitat Politècnica de València. | spa |
dc.relation.references | Casado-Mancebo, M. (2021). Una aproximación a la lingüística computacional. Revista de Filosofía, Letras y Humanidades,, 746–761. | spa |
dc.relation.references | Casanova, E., Gölge, E., Meyer, J., davis, k., & Morais, R. (29 de septiembre de 2022). Coqui. Obtenido de Make the impossible possible and the painful painless with Coqui: https://coqui.ai/ | spa |
dc.relation.references | Celis Nuñez, J. D. (2017). Modelo Acústico y de Lenguaje del Idioma Español para el dialecto Cucuteño, Orientado al Reconocimiento Automático del Habla. Ingeniería, 22(3), 362. | spa |
dc.relation.references | Churbanov, A., & Winters-Hilt, S. (2008). Implementing EM and Viterbi algorithms for Hidden Markov Model in linear memory. BMC Bioinformatics 9, 224. | spa |
dc.relation.references | Colompar, B. C. (2018). Desarrollo de un sistema de Reconocimiento Automático del Habla en Rumano para el subtitulado de vídeos educativos. Valencia: Escola Tècnica Superior d’Enginyeria Informàtica Universitat Politècnica de Valencia. | spa |
dc.relation.references | Cuomo, J. (2013). Mobile app development, JavaScript. IBM Software. | spa |
dc.relation.references | de Luna, E. B., & Expósito López, J. (2011). UNIDAD 3. EL PROCESO DE INVESTIGACIÓN EDUCATIVA II: INVESTIGACIÓN-ACCIÓN. FACULTAD DE CIENCIAS DE LA EDUCACIÓN - UNIVERSIDAD DE GRANADA, 35-50. | spa |
dc.relation.references | Deng, L., & Yu, D. (2014). Deep Learning: Methods and Applications. En M. d. Jongh, Foundations and Trends R© in Signal Processing Vol. 7 (págs. 197-387). | spa |
dc.relation.references | Doshi, K. (25 de Mar de 2021). Audio Deep Learning Made Simple: Automatic Speech Recognition (ASR), How it Works. Obtenido de Speech-to-Text algorithm and architecture, including Mel Spectrograms, MFCCs, CTC Loss and Decoder, in Plain English: https://towardsdatascience.com/audio-deep-learning-made-simple-automatic-speech-recognition-asr-how-it-works-716cfce4c706 | spa |
dc.relation.references | Emilio, M. D. (2015). Embedded Systems Design for High-Speed Data Acquisition and Contro. Springer. | spa |
dc.relation.references | Enciclopedia de Ejemplos. (29 de Septiembre de 2022). Tipos de acentos. Obtenido de Cuáles son los Tipos de acentos: https://www.ejemplos.co/tipos-de-acentos/ | spa |
dc.relation.references | Ferrucci, D., Levas, A., Bagchi, S., Gondek, D., & Mueller, E. T. (2013). Watson: Beyond Jeopardy! Science Direct, 93-105. | spa |
dc.relation.references | Field, C. (31 de Agosto de 2021). Towards Data Science. Obtenido de Hidden Markov Models: an Overview: https://towardsdatascience.com/hidden-markov-models-an-overview-98926404da0e | spa |
dc.relation.references | Gemmeke, J. F., Ellis, D., Freedman, D., Jansen, A., Lawrence, W., Moore, R. C., . . . Ritter, M. (2017). Audio Set: An ontology and human-labeled dataset for audio events. 2017 IEEE International Conference on Acoustics, Speech and Signal Processing (ICASSP) (págs. 776 - 780). New Orleans: IEEE. | spa |
dc.relation.references | Google. (15 de 07 de 2022). Google Kubernetes Engine. Obtenido de GKE: https://Cloud.Google.com/kubernetes-engine | spa |
dc.relation.references | Google Cloud. (3 de Mayo de 2022). Pricing | Cloud speech-to-text | Google Cloud. Obtenido de https://Cloud.Google.com/speech-to-text/pricing | spa |
dc.relation.references | Google Cloud. (26 de abril de 2022). Speech-to-Text: Automatic Speech Recognition. Obtenido de https://Cloud.Google.com/speech-to-text | spa |
dc.relation.references | Hannun, A., Case, C., Caspe, J., Catanzaro, B., Diamos, G., Elsen, E., . . . Ng, A. Y. (2014). Deep Speech: Scaling up end-to-end speech recognition. Baidu Research Silicon Valley AI Lab, 12. Obtenido de arXiv:1412.5567. | spa |
dc.relation.references | Hashemnia, S. &. (2021). Human EEG and Recurrent Neural Networks Exhibit Common Temporal Dynamics During Speech Recognition. Frontiers in Systems Neuroscience, 617605. | spa |
dc.relation.references | Haubold, A., & Kender, J. (2007). Alignment of Speech to Highly Imperfect Text Transcriptions. 2007 IEEE International Conference on Multimedia and Expo (págs. 224 - 227). Beijing: IEEE. | spa |
dc.relation.references | Herzog, O. (2005). Applied Wearable Computing, IFAWC. 2nd International Forum on Applied Wearable Computing, IFAWC: Proceedings, March 17-18, 2005 in Zurich, Switzerland (pág. 188). Zurich, Switzerland: VDE Verlag. | spa |
dc.relation.references | IBM. (26 de Abril de 2022). Watson Speech to Text. Obtenido de Convert speech into text using AI-powered speech recognition and transcription: https://www.ibm.com/Cloud/watson-speech-to-text | spa |
dc.relation.references | Internet Engineering Task Force. (9 de 12 de 2011). The WebSocket Protocol. Obtenido de Internet Engineering Task Force: https://datatracker.ietf.org/doc/html/rfc6455 | spa |
dc.relation.references | Lecorvé, G. (25 de Jul de 2022). Automatic speech recognition. Obtenido de Vocal and Acoustic Interactions - Automatic Speech Recognition : http://people.irisa.fr/Gwenole.Lecorve/lectures/ASR.pdf | spa |
dc.relation.references | Lee, A., Kawahara, T., & Shikano, K. (2001). Julius — an Open Source Real-Time Large Vocabulary Recognition Engine. 7th European Conference on Speech Communication and Technology (págs. 1-4). Scandinavia: INTERSPEECH. | spa |
dc.relation.references | Li, X., Sun, J., Lei, X., Zou, W., & Zhao, S. (22 de septiembre de 2022). Athena. Obtenido de What is Athena?: https://athena-team.readthedocs.io/en/latest/introduction/introduction.html | spa |
dc.relation.references | Lunden, I. (24 de Enero de 2013). techcrunch. Obtenido de Amazon Gets Into Voice Recognition: https://techcrunch.com/2013/01/24/amazon-gets-into-voice-recognition-buys-ivona-software-to-compete-against-apples-siri/ | spa |
dc.relation.references | Mahmood, A., & Köse, U. (15 de Enero de 2021). Speech recognition based on Convolutional neural networks and MFCC algorithm. Advances in Artificial Intelligence Research (AAIR), 6-12. | spa |
dc.relation.references | Mateus, E. O. (2008). HIDDEN MAKROV MODELS (HMM'S) Y APLICACIONES. Cartagena de indias D.T y C: Universidad Tecnologica de Bolivar. | spa |
dc.relation.references | Ming, Z., Nan, D., Shujie, L., & Heung-Yeung, S. (Marzo de 2020). Progress in Neural NLP: Modeling, Learning, and Reasoning. (M. R. Asia, Ed.) Engineering Volume 6, Issue 3, 275-290. | spa |
dc.relation.references | Ministerio de Educación. (23 de Marzo de 2020). Decreto 457 mediante el cual se imparten instrucciones para el cumplimiento del Aislamiento Preventivo Obligatorio. Obtenido de mineducacion.gov.co: https://www.mineducacion.gov.co/1759/w3-printer-394357.html | spa |
dc.relation.references | Mohamed, A. r. (2014). Deep Neural Network acoustic models for ASR. Toronto: Department of Computer Science University of Toronto. | spa |
dc.relation.references | Mozilla. (15 de 08 de 2022). Discourse Mozilla. Obtenido de Deep Speech forum: https://discourse.mozilla.org/c/deepspeech/247 | spa |
dc.relation.references | Mozilla. (10 de febrero de 2022). Mozilla Voice. Obtenido de How we're making Common Voice even more linguistically inclusive: https://foundation.mozilla.org/en/blog/how-we-are-making-common-voice-even-more-linguistically-inclusive/ | spa |
dc.relation.references | Mozilla Corporation. (21 de Septiembre de 2022). Mozilla Common Voice. Obtenido de Common Voice: https://commonvoice.mozilla.org/es/criteria | spa |
dc.relation.references | Naik, S., Naik, N., Prabhu, G., Bhayje, A., Naik, V. P., & Aswale, S. (9 de junio de 2021). A Survey on different approaches for Speech to Text and Text to Speech in Email System for Visually Impaired People. International Journal of Computer Applications (volume 183 – No. 9), 20-23. | spa |
dc.relation.references | Ortega, S. V. (1999). Sobre las relaciones de la morfologia con la sintaxis. Revista Española de Lingüistica, 257-2781. | spa |
dc.relation.references | Povey, D. (10 de Mayo de 2022). Kaldi. Obtenido de https://kaldi-asr.org/doc/ | spa |
dc.relation.references | Pratap, V., Hannun, A., Xu, Q., Cai, J., Kahn, J., Synnaeve, G., . . . Collobert, R. (2019). wav2letter++: The Fastest Open-source Speech Recognition System. ICASSP 2019 (págs. 6460-6464). Brighton, UK : IEEE. | spa |
dc.relation.references | Reactjs. (15 de Septiembre de 2022). Refs and the DOM. Obtenido de Refs provide a way to access DOM nodes or React elements created in the render method.: https://reactjs.org/docs/refs-and-the-dom.html | spa |
dc.relation.references | Reyzábal Manso, M. I. (2005). Modelos de lenguaje y tecnología del habla. Recuperado el 15 de Septiembre de 2022, de Educación XX1 2005, 8 ( ): https://www.redalyc.org/articulo.oa?id=70600806 | spa |
dc.relation.references | Sadeen , A., Muna, A., Alanoud, A., Turkiayh, A., Raghad, A., Rimah, A., . . . Maha, A. (14 de september de 2021). Automatic Speech Recognition: Systematic Literature Review. IEEE Access, 131858 - 131876. | spa |
dc.relation.references | Sean, W. (29 de noviembre de 2017). Mozilla Press Center. Obtenido de Announcing the Initial Release of Mozilla’s Open Source Speech Recognition Model and Voice Dataset: https://blog.mozilla.org/press/2017/11/announcing-the-initial-release-of-mozillas-open-source-speech-recognition-model-and-voice-dataset/ | spa |
dc.relation.references | Shivangi, N., & Ashika, J. (2020). A REVIEW ON METHODS FOR SPEECH-TO-TEXT AND TEXT-TO-SPEECH. International Research Journal of Engineering and Technology, 6. | spa |
dc.relation.references | Significados. (16 de Enero de 2020). Obtenido de https://www.significados.com/marco-conceptual/ | spa |
dc.relation.references | Smith, D. R. (2003). Digital Transmission Systems. Springer. | spa |
dc.relation.references | The International Phonetic Association. (25 de Septiembre de 2022). Reproduction of The International Phonetic Alphabet. Obtenido de https://web.archive.org/web/20121010121927/http://www.langsci.ucl.ac.uk/ipa/ipachart.html | spa |
dc.relation.references | The World Wide Web Consortium (W3C). (15 de 05 de 2006). Understanding the New Language Tags. Obtenido de WC3 Internationalization: https://www.w3.org/International/articles/bcp47/ | spa |
dc.relation.references | Trivedi, A., Pant, N., Shah, P., & Sonik, S. (2018). Speech to text and text to speech recognition systems-Areview. En N. Pant, IOSR Journal of Computer Engineering (IOSR-JCE) Volume 20, Issue 2, Ver. I (págs. 38-39). Mumbai: NMIMS University. | spa |
dc.relation.references | Trmal, J. ". (8 de mayo de 2022). openslr. Obtenido de About OpenSLR: https://www.openslr.org/ | spa |
dc.relation.references | Vivek, B., Sashi, B., Virender, K., & Vinay, K. (2020). Development of Robust Automatic Speech Recognition System for Children's using Kaldi Toolkit. 2020 Second International Conference on Inventive Research in Computing Applications (ICIRCA) (págs. 10-13). Coimbatore,India: IEEE. | spa |
dc.relation.references | Vivek, C. V. (18 de Agosto de 2020). Markov and Hidden Markov Model. Obtenido de Elaborated with examples: https://towardsdatascience.com/markov-and-hidden-markov-model-3eec42298d75 | spa |
dc.relation.references | Vu, T. N. (2014). Automatic Speech Recognition for Low-resource Languages and Accents Using Multilingual and Crosslingual Information. Karlsruhe Germany: Karlsruhe Institute of Technology KIT. | spa |
dc.relation.references | Wikipedia. (18 de mayo de 2022). Wikcionario . Obtenido de https://es.wikipedia.org/wiki/Wikcionario | spa |
dc.relation.references | Yalta, N., Hayashi, T., & Yalta, N. (10 de septiembre de 2022). ESPnet: . Obtenido de end-to-end speech processing toolkit: https://github.com/espnet/espnet | spa |
dc.rights | Attribution-NonCommercial 4.0 International | eng |
dc.rights.accessrights | info:eu-repo/semantics/openAccess | spa |
dc.rights.coar | http://purl.org/coar/access_right/c_abf2 | spa |
dc.subject.proposal | Voz a texto | spa |
dc.subject.proposal | Software Libre | spa |
dc.subject.proposal | Educación Telepresencial | spa |
dc.subject.proposal | Discapacidad auditiva | spa |
dc.subject.proposal | Inteligencia Artificial | spa |
dc.subject.proposal | Voice to text | eng |
dc.subject.proposal | Open Source Software | eng |
dc.subject.proposal | Virtual Education | eng |
dc.subject.proposal | Hearing Impaired | eng |
dc.subject.proposal | Markov Chains | eng |
dc.subject.proposal | Redes neuronales convulsionadas | spa |
dc.subject.proposal | Cadenas de Markov | spa |
dc.subject.proposal | Automated Subtitles | eng |
dc.subject.proposal | Subtitulos Automatizados | spa |
dc.subject.proposal | Convolutional neural network | spa |
dc.title | Creación de una herramienta de voz a texto utilizando un motor de software libre para facilitar la inclución digital en educacion telepresencial a la comunidad no oyente de la Universidad ECCI | spa |
dc.type | Trabajo de grado - Pregrado | spa |
dc.type.coar | http://purl.org/coar/resource_type/c_7a1f | spa |
dc.type.coarversion | http://purl.org/coar/version/c_970fb48d4fbd8a85 | spa |
dc.type.content | Text | spa |
dc.type.driver | info:eu-repo/semantics/bachelorThesis | spa |
dc.type.redcol | https://purl.org/redcol/resource_type/TP | spa |
dc.type.version | info:eu-repo/semantics/updatedVersion | spa |
dspace.entity.type | Publication |
Archivos
Bloque original
1 - 4 de 4
Cargando...
- Nombre:
- Trabajo de grado.pdf
- Tamaño:
- 2.4 MB
- Formato:
- Adobe Portable Document Format
- Descripción:
Cargando...
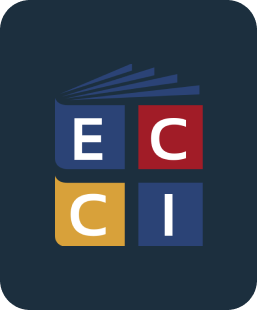
- Nombre:
- Acta de opción de grado.pdf
- Tamaño:
- 215.1 KB
- Formato:
- Adobe Portable Document Format
- Descripción:
Cargando...
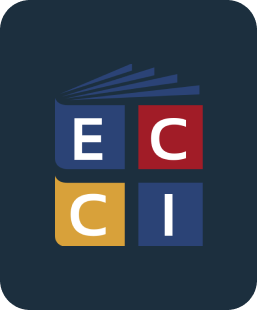
- Nombre:
- Cesión de derechos.pdf
- Tamaño:
- 976.09 KB
- Formato:
- Adobe Portable Document Format
- Descripción:
Bloque de licencias
1 - 1 de 1
Cargando...
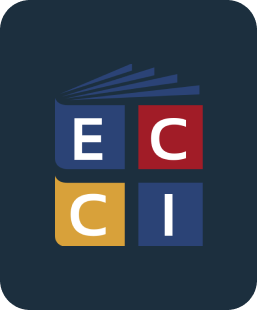
- Nombre:
- license.txt
- Tamaño:
- 14.45 KB
- Formato:
- Item-specific license agreed upon to submission
- Descripción: